The CSAT metric used in customer support has existed in its current form since the late 1980s and early 1990s. Despite the fact that over that time the widespread adoption of the internet and email has occurred, AI chatbots are being adopted, customer expectations have drastically evolved, and call center automation is growing exponentially, CSAT continues to be used almost 40 years later as a benchmark for customer experience. CSAT is no longer a meaningful or actionable representation of your customers’ experiences with your brand.
The Flaws in CSAT
CSAT as a measure of CX has a few major flaws. The first and most important is, at best, it is a lagging indicator of your customer sentiment. Even in a world where 100% of CSAT surveys were filled out, by then it is too late to improve the experience. In reality, it is well known that completion rates tend to be much lower with industry benchmarks between 4% and 15%. And of those surveys, most are a simple “thumbs up/thumbs down” or “1-5 scale” survey that does not provide actionable insight into what you could have done better. That lack of context means that even if the trend is down, it is hard to pinpoint what product, process, or service needs to change to improve.
In addition, should AI agents or resolutions through AI ticketing be measured in the same way as human agents? With contact center automation on the rise, CX leaders are seeing a difference between the mistakes humans make versus the ones AI agents or even the best AI chatbots make. CSAT is not equipped to make these important distinctions.
With low response rates, missing context, the delay in aggregation, and other factors such as a cultural tendency to use or not use extreme ratings in a consistent manner, CSAT is less useful in today’s customer experience landscape
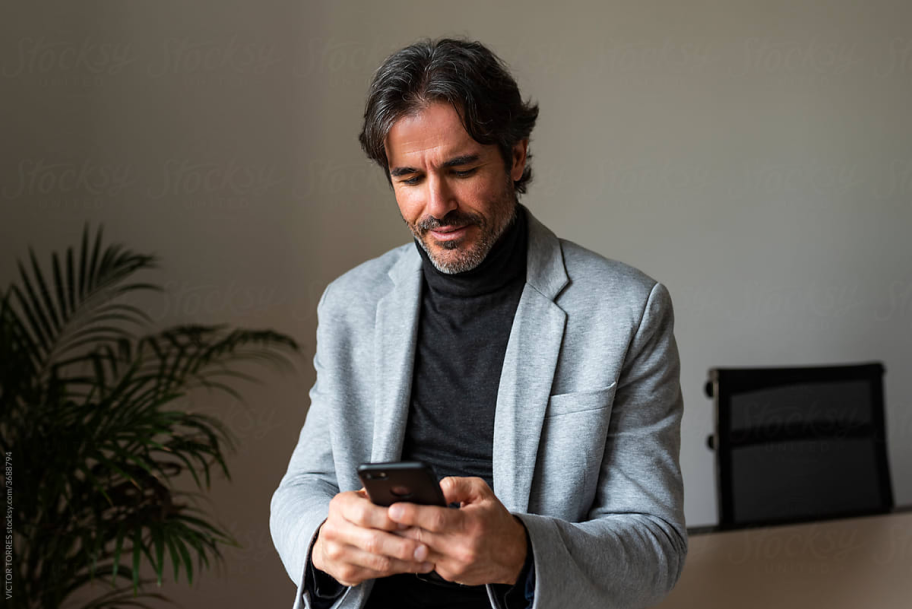
Beyond Agent CSAT
As Steve Jobs is quoted as saying, “Get closer than ever to your customers. So close that you tell them what they need well before they realize it themselves.” CSAT as a metric lags in this capability. With the ongoing expansion of LLMs, AI chatbots, and other Generative AI models that are happening, it is easier than ever to achieve this goal. Here are a few ways you can go beyond CSAT.
Understand Value Drivers and Expectations
What do your customers value? For example, a recent study of drive-thrus at top fast food chains found that while Chick-fil-A’s wait time was the highest of all their competitors, their customers were most satisfied and their orders were most accurate. Their customers valued order accuracy and food quality over wait time. Without knowing that, a CX leader could easily have made the decision to reduce wait time, which would ultimately worsen customer satisfaction.
For this reason, you need to deeply understand why your customers want your product or service. For example, measuring Value Enhancement Score (VES), which Gartner states is “far more of a leading indicator, enabling service leaders to more accurately predict a customer’s future loyalty.” With VES, you measure the customers confidence in your product or service and if they are gaining the value expected from it.
For example, if your customers use your product outside of your operating hours for support, they may highly value self-help through a robust knowledge base, a well tuned workflow automation, or a conversational AI agent.
Reduce the Friction
The next step is to figure out where you are adding friction in your processes. Are you making it difficult for customers or prospects to gain the value they expect? Studying web navigation and product usage behaviors to see where users are stalling, or out tight abandoning a process. Shadow or evaluate real users, not mocked up tests in your QA or web development team. You can also use Customer Effort Score (CES) as a metric to help understand how difficult a customer found a particular process.
Another way to think about reducing friction is to create a conversational AI agent to answer questions about your pricing, product, company profile, policies, compliance, store hours, etc. Overall, the goal here is to deliver the value you know they want with as little effort as possible on their behalf.
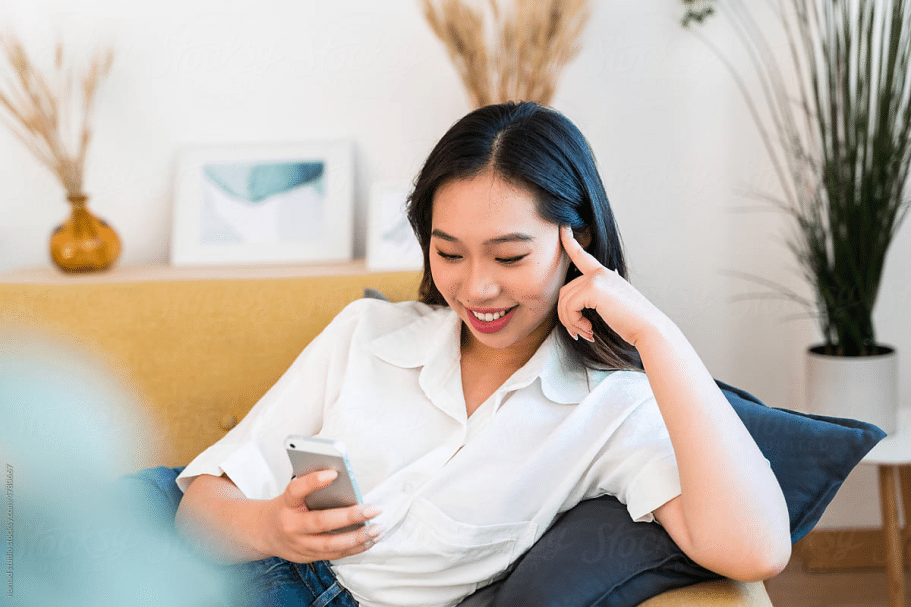
“The best AI chatbots will bring answers to your customers in an intuitive way and reduce their need to search or navigate for common information.”
Improve AI Insight and Analytics
The single biggest improvement to CSAT you can make as part of your contact center automation strategy is with a full contextual understanding of what your customers and prospects truly think. Combining the explicit feedback you receive through surveys, support tickets, social media posts, communities, etc. with existing data you collect such as product usage patterns, industry, contract value etc. you can use an AI analytics tool to deeply understand customer sentiment.
A great way to improve upon your existing metrics is to pair them with insights you gathered. For example, if your churn rate has risen, instead of reporting just that metric and one or two small data points from an exit-survey you conduct, look across all sentiment and see if a particular product change, brand image, or market force has swayed your customers elsewhere. That is far more powerful than a churn percentage alone.
These insights will help you to create more prescriptive projects. Using the data to support investment in an area of your product or service, which customer profile will be impacted the most, and allow you to be more confident in your ROI projections. Similarly you can use this data to tune your AI agents or AI chatbot to meet more customer needs or enhance the automations that already exist